Historical bias in AI systems
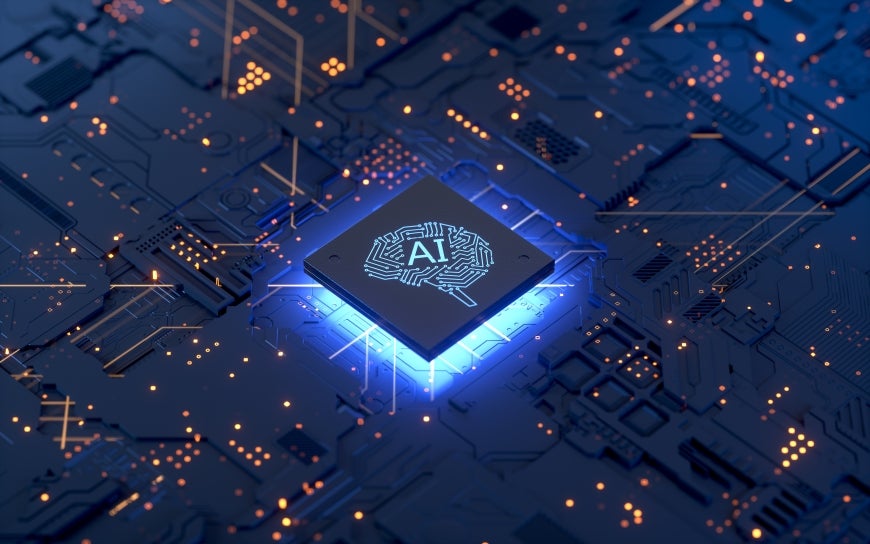
Published:
Topic(s): Technology, Privacy and Data, Artificial Intelligence, Federal Discrimination Law
The example shown below is fictional but based on the types of scenarios that are known to occur in real life. You can learn more about addressing algorthmic bias to ensure ethical AI by reading our Technical Paper.
Ensuring ethical AI
Artificial intelligence (AI) systems are trained using data. AI systems learn patterns in the data and then make assumptions based on that data that can have real-world consequences.
For example, if the training data shows a higher prevalence of suitable individuals in one group versus another, an AI system trained on that data will prefer candidates from that group when selecting people.
Women face a ‘gender pay gap’, barriers to leadership roles in the workplace, and experience reduced employment opportunities due to family and caring responsibilities.
These structural issues mean women are less likely to earn as much as men. If an individual’s income is an important factor in determining their suitability as a customer of a particular service, an AI system tasked with selecting future customers would likely exhibit preferential treatment towards men.
Historical bias
Historical bias arises when the data used to train an AI system no longer accurately reflects the current reality.
For example while the ‘gender pay gap’ is still a problem, historically, the financial inequality faced by women was even worse.
For people using screen readers: the graph below shows the gender pay gap reducing over time from 24.7% in 2014 to 20.8% in 2019 (total remuneration) and from 19.9% in 2014 to 15.5% in 2019 (base salary). As the gender pay gap narrows, the gap in customer suitability between men and women also becomes less significant.
INFOGRAPHIC: Shifting Trends
Slider infographic showing rejections due to historical bias
When the AI system is trained using historical data, the system rejects more women as potential customers (the gender pay gap has reduced since 2014 but the AI system is making decisions based on out-of-date income data from 2014).
The more out-of-date the data, the more women the AI system rejects as ‘unsuitable’. In some circumstances, this could be considered unlawful discrimination under the Sex Discrimination Act.
Move the slider in the infographic below to compare what happens with recent and out-of-date data.
For people using screen readers: the infographic below shows a comparison of the number of people rejected by an AI system when it is trained using newer or older data sets.
The infographic has 20 men and 20 women (all potential customers). If the AI system uses data from 2015, it rejects 3 women in 20 due to historical bias. If the AI system uses data from 2017 it rejects 2 women in 20 due to historical bias. When it uses data from 2019, it rejects 1 man and 1 woman due to insufficient training data. More on this below.
Mitigation
Did you notice that when you move the slider on the infographic above to 2018/20 it says ‘Rejected due to insufficient training data”?
This shows that sometimes using only the latest data can also cause issues because using a smaller data set can mean there is not enough data to make accurate decisions. This means using only the most recent data available is not always a ‘magic bullet’ to remove algorithmic bias.
Other companies have tried to get around the issue of algorithmic bias by removing a person’s gender from the data set. But there is often enough other information in the data set for the AI system to identify someone as male or female.
For example, browsing history or financial transaction data can strongly correlate with a person’s gender, so when gender is removed from the data set, the AI system could instead use these (among many other sources of information) as proxies for gender, and downrank individuals who visit websites or buy products that are popular with women.
Although some men may show similar patterns (visiting websites and buying products typically associated with women) and consequently also be downranked, overall women would still be more likely to be disadvantaged by the AI system than men.
Therefore, removing the ‘protected attribute’ (gender) from the data set doesn’t mitigate algorithmic bias in these circumstances.
For people using screen readers: the diagram above shows potential mitigations and considerations for addressing bias in AI.
- Gather more appropriate data (the considerations are privacy and the fact this may be impractical)
- Adjust the selection criteria for the disadvantaged group (need to consider this may lead to a reduction in the system’s accuracy and increase the number of false positives in the disadvantaged group)
- Train the AI system to incorporate fairness into its decisions (considerations are the same as for #2).